Difference between revisions of "PCL/OpenNI tutorial 5: 3D object recognition (pipeline)"
m |
m |
||
(4 intermediate revisions by the same user not shown) | |||
Line 25: | Line 25: | ||
** [http://www.pointclouds.org/assets/uploads/PCL-IAS13_Recognition.pdf 3D Object Recognition in Clutter with the Point Cloud Library] | ** [http://www.pointclouds.org/assets/uploads/PCL-IAS13_Recognition.pdf 3D Object Recognition in Clutter with the Point Cloud Library] | ||
* '''Publication''': | * '''Publication''': | ||
− | ** [ | + | ** [https://www.inf.ethz.ch/personal/zeislb/publications/aldoma_2012jram_PCLTutorial.pdf Point Cloud Library: Three-Dimensional Object Recognition and 6 DoF Pose Estimation] (Aitor Aldoma et al., 2012) |
</div> | </div> | ||
Line 37: | Line 37: | ||
− | Another possibility is to do it virtually, rendering 3D models of the objects (modelled beforehand with CAD software like [ | + | Another possibility is to do it virtually, rendering 3D models of the objects (modelled beforehand with CAD software like [https://en.wikipedia.org/wiki/Blender_%28software%29 Blender]) and using the Z buffer, which contains the depth information, to simulate the input a depth sensor would give. The perks of doing this include: no segmentation step is necessary to get the object cluster, and the ground truth pose information will be 100% exact. |
Line 56: | Line 56: | ||
==Getting a full model== | ==Getting a full model== | ||
− | Like I explained above, getting a full .pcd model for our object with a sensor requires some careful setup, good segmentation, normal computation and possibly resampling. On the other hand, if we use a 3D CAD model, it becomes much easier. A program like [ | + | Like I explained above, getting a full .pcd model for our object with a sensor requires some careful setup, good segmentation, normal computation and possibly resampling. On the other hand, if we use a 3D CAD model, it becomes much easier. A program like [https://en.wikipedia.org/wiki/Blender_%28software%29 Blender] (free and open source) can be used to design a model of the object, after taking some measurements. Programs like [https://en.wikipedia.org/wiki/MeshLab MeshLab] can convert to and from many types of 3D formats. In this case, the one favored by PCL is binary [https://en.wikipedia.org/wiki/PLY_%28file_format%29 PLY] with normals (Blender can export to ASCII PLY, but I have sometimes encountered a processing error with those). |
===Raytracing=== | ===Raytracing=== | ||
Line 66: | Line 66: | ||
<syntaxhighlight lang=Bash>pcl_mesh2pcd <input.ply> <output.pcd></syntaxhighlight> | <syntaxhighlight lang=Bash>pcl_mesh2pcd <input.ply> <output.pcd></syntaxhighlight> | ||
− | Here you can see the result of converting a mesh exported from Blender, the program's mascot, [ | + | Here you can see the result of converting a mesh exported from Blender, the program's mascot, [https://en.wikipedia.org/wiki/Blender_%28software%29#Suzanne Suzanne]: |
Line 103: | Line 103: | ||
===Code=== | ===Code=== | ||
− | You can customize the snapshot generation process by making direct use of the <span style="color:#FF1493">"RenderViewsTesselatedSphere"</span> class, which is what the previous tool uses internally. The code is the following: | + | You can customize the snapshot generation process by making direct use of the <span style="color:#FF1493">"RenderViewsTesselatedSphere"</span> class, which is similar to what the previous tool uses internally. The code is the following: |
<syntaxhighlight lang=CPP>#include <pcl/io/vtk_lib_io.h> | <syntaxhighlight lang=CPP>#include <pcl/io/vtk_lib_io.h> | ||
Line 182: | Line 182: | ||
===ISS=== | ===ISS=== | ||
− | Another keypoint detector available in PCL is the ISS (Intrinsic Shape Signatures) one. ISS is a local descriptor presented in 2009, which creates a view-independent signature of the local surface patch. An algorithm for choosing keypoints was also included to better fit the descriptor. The algorithm scans the surfaces and chooses only points with large variations in the [ | + | Another keypoint detector available in PCL is the ISS (Intrinsic Shape Signatures) one. ISS is a local descriptor presented in 2009, which creates a view-independent signature of the local surface patch. An algorithm for choosing keypoints was also included to better fit the descriptor. The algorithm scans the surfaces and chooses only points with large variations in the [https://en.wikipedia.org/wiki/Principal_curvature principal direction] (the shape of the surface), which are ideal for keypoints. |
You can use the associated PCL class this way: | You can use the associated PCL class this way: | ||
Line 424: | Line 424: | ||
===Hough voting=== | ===Hough voting=== | ||
− | This method is a bit more complex. It uses a technique known as [ | + | This method is a bit more complex. It uses a technique known as [https://en.wikipedia.org/wiki/Hough_transform Hough transform], which was originally devised to perform line detection on 2D images, though it was later expanded to work with arbitrary shapes or higher dimensions. The method works with a voting procedure, with votes being cast on candidates that are found to be better suitable. If enough votes are accumulated for a given position in the space, then the shape is considered "found" there and its parameters are retrieved. |
The voting procedure is carried out in a parameter space, which would have 6 dimensions in case a full pose needed to be estimated (rotation plus translation). Because that would be computationally expensive, the method implemented in PCL uses only a 3D Hough space, and employs local Reference Frames (RFs) to account for the remaining 3 DoF. Its computation can then be divided in two steps. | The voting procedure is carried out in a parameter space, which would have 6 dimensions in case a full pose needed to be estimated (rotation plus translation). Because that would be computationally expensive, the method implemented in PCL uses only a 3D Hough space, and employs local Reference Frames (RFs) to account for the remaining 3 DoF. Its computation can then be divided in two steps. | ||
Line 858: | Line 858: | ||
==Matching== | ==Matching== | ||
− | Like with the local pipeline, once all the descriptors have been computed we have to perform a search for their nearest neighbors in the database. The [ | + | Like with the local pipeline, once all the descriptors have been computed we have to perform a search for their nearest neighbors in the database. The [https://en.wikipedia.org/wiki/Taxicab_geometry Manhattan or L1 distance] is recommended over the [https://en.wikipedia.org/wiki/Euclidean_distance Euclidean or L2] one because it is more robust to occlusions. |
Usually, more than one neighbor is retrieved, which means that the found object's pose is somewhere between the two. The next steps can help improving the accuraccy of the estimation. | Usually, more than one neighbor is retrieved, which means that the found object's pose is somewhere between the two. The next steps can help improving the accuraccy of the estimation. | ||
Line 869: | Line 869: | ||
==Pose estimation== | ==Pose estimation== | ||
− | The object's position can be determined by computing and aligning the [[PCL/OpenNI_tutorial_2:_Cloud_processing_(basic)#Computing_the_centroid|centroids]] of the clusters (the one in the scene, and the one in the snapshot). For the rotation, we must use the viewpoint information encoded in the descriptor. This still leaves the roll angle unresolved, as global descriptors are invariant to it. Now is when the Camera Roll Histogram ([[PCL/OpenNI_tutorial_5:_3D_object_recognition_%28pipeline%29#CRH|CRH]]) that we should have stored next to the descriptor comes in handy. The angle can be obtained by aligning the current CRH with the stored one, completing the 6 DoF pose estimation. | + | The object's position can be determined by computing and aligning the [[PCL/OpenNI_tutorial_2:_Cloud_processing_(basic)#Computing_the_centroid|centroids]] of the clusters (the one in the scene, and the one in the snapshot). For the rotation, we must use the viewpoint information encoded in the descriptor (you can check the ground truth pose information that was saved with the matched snapshot). This still leaves the roll angle unresolved, as global descriptors are invariant to it. Now is when the Camera Roll Histogram ([[PCL/OpenNI_tutorial_5:_3D_object_recognition_%28pipeline%29#CRH|CRH]]) that we should have stored next to the descriptor comes in handy. The angle can be obtained by aligning the current CRH with the stored one, completing the 6 DoF pose estimation. |
===CRH=== | ===CRH=== | ||
Line 953: | Line 953: | ||
==Pose refinement== | ==Pose refinement== | ||
− | The 6 DoF pose estimation can be refined with the use of the [ | + | The 6 DoF pose estimation can be refined with the use of the [[PCL/OpenNI_tutorial_3:_Cloud_processing_%28advanced%29#Iterative_Closest_Point_.28ICP.29|Iterative Closest Point]] (ICP) algorithm. It will try to continuously realign the clouds to improve the transformation, until the termination condition is met (maximum iterations, and distance or error threshold). This is identical to performing [[PCL/OpenNI_tutorial_3:_Cloud_processing_%28advanced%29#Registration|registration]], only between input and model. |
==Hypothesis verification== | ==Hypothesis verification== |
Latest revision as of 23:26, 1 November 2015
Go to root: PhD-3D-Object-Tracking
In our previous article we saw how 3D descriptors could be used to identify points or clusters. But in order to have a working object recognition system, many more things are necessary. The sequence of steps that we have to implement to make such a system is known as the pipeline. This final article will explain how to do it. The scope of what we will talk about is very wide and many has been written, so you should consider this a simple introductory tutorial, to build a basic knowledge so you can experiment further.
Overview
Ideally, a 3D object recognition system should be able to grab clouds from the device, preprocess them, compute descriptors, compare them with the ones stored in our object database, and output all matches with their position and orientation in the scene, in real time. Several components must then be implemented to perform these sequential steps, each one taking as input the output of the previous. This pipeline will be different depending on what type of descriptor we are using: local or global.
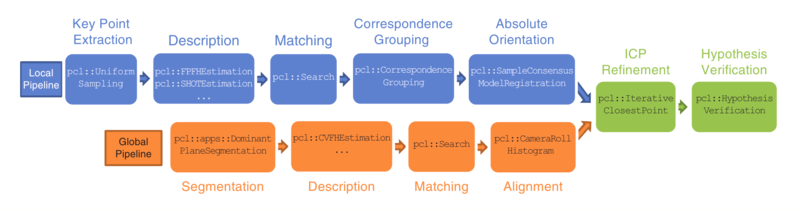
First of all, we have to train the system. Training means, in this case, creating a database will all the objects we want to be able to recognize, and the descriptors for every associated pose. Only after that we can implement the recognition pipeline. Also, there are some postprocessing steps that are not mandatory to perform but will yield better results if done, like pose refinement and hypothesis verification.
In the next sections we will go over every step, with some PCL code snippets, as always.
- Tutorials:
- Publication:
- Point Cloud Library: Three-Dimensional Object Recognition and 6 DoF Pose Estimation (Aitor Aldoma et al., 2012)
Training
As stated, we require a database with all the objects we intend to recognize later (it is possible to build a recognition system that works with object categories and high level descriptions, for example to find all things that "look" like a chair in the scene, but that is an entirely different matter). The most common way to do this is to take dozens of snapshots of every object from different angles. This can be done with a device such as a pan-tilt unit (which consists of a mechanical platform that can be rotated on two axes in known, precise amounts), or with a common table and a printed checkerboard pattern on it, to be used as ground truth to get the camera position and orientation. Of course, you need a physical copy of the object first.
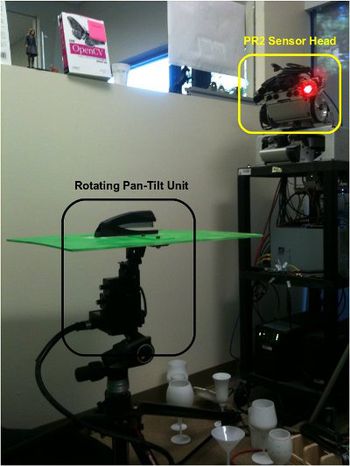
Another possibility is to do it virtually, rendering 3D models of the objects (modelled beforehand with CAD software like Blender) and using the Z buffer, which contains the depth information, to simulate the input a depth sensor would give. The perks of doing this include: no segmentation step is necessary to get the object cluster, and the ground truth pose information will be 100% exact.
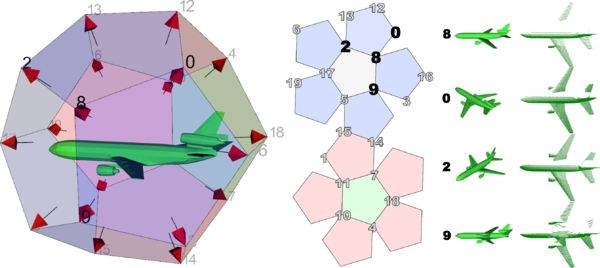
Many 3D object datasets that are available for download have been created with one of these methods. Whichever you choose, be sure to be consistent and take all snapshots uniformly, with the same sampling level (one every X degrees). When choosing this amount, try to make a compromise between the complexity of the database (size is usually not a problem, but the more snapshots you have, the longer it will take to finish the matching step) and the precision of pose estimation.
After this, the desired descriptor(s) must be computed for every snapshot of each object, and saved to disk. If global descriptors are being used, a Camera Roll Histogram (CRH) should be included in order to retrieve the full 6 DoF pose, as many descriptors are invariant to the camera roll angle, which would limit the pose estimation to 5 DoF. Finally, ground truth information about the camera position and orientation will make it possible to compare it against the result given back by the recognition system. Most datasets include such information in text files next to the corresponding snapshot, usually in the form of a 3x3 rotation matrix and a 3D translation vector.
If you are using local descriptors, it is possible to work with a database of full objects, instead of partial snapshots (this can not be done with global descriptors because they are computed from the whole cluster, and a full object is something we would never get in practice; plus, they also encode viewpoint information). To do this, you would have to capture the object from all angles and then use registration or other technique to stitch the clouds together in order to create a complete, continous model. Resampling should also be needed because overlapping areas would have higher point density. Also, as normals are oriented according to the viewpoint when they are computed, you would have to merge them properly so they all point outwards, instead of recomputing them after composing the object cluster. The perk of using full objects is that the matching step will be shorter, as the system will not have to check against the descriptors of multiple snapshots.
Getting a full model
Like I explained above, getting a full .pcd model for our object with a sensor requires some careful setup, good segmentation, normal computation and possibly resampling. On the other hand, if we use a 3D CAD model, it becomes much easier. A program like Blender (free and open source) can be used to design a model of the object, after taking some measurements. Programs like MeshLab can convert to and from many types of 3D formats. In this case, the one favored by PCL is binary PLY with normals (Blender can export to ASCII PLY, but I have sometimes encountered a processing error with those).
Raytracing
PCL offers a couple of command line tools to render a .pcd cloud file from a CAD model. The first one does this by raytracing. It uses a 3D sphere or icosahedron that is tesselated (divided in polygonal regions). Then, the virtual camera is placed in each of the vertices (or the polygons' centers) pointing to the origin, where the object model is. Multiple snapshots are rendered, and the final cloud is composed from this information.
You can invoke it like this:
pcl_mesh2pcd <input.ply> <output.pcd>
Here you can see the result of converting a mesh exported from Blender, the program's mascot, Suzanne:
The tool has some parameters available, you can check their usage with:
pcl_mesh2pcd -h
For example, one of the parameters controls the size of the voxel grid used to downsample the cloud after the raytracing operation. With this you can regulate the point density of the resulting .pcd file.
Sampling
The second tool we are going to see employs sampling, with a voxel grid. It gets similar results to the previous one. You can invoke it in an identical way:
pcl_mesh_sampling <input.ply> <output.pcd>
It has the same parameters as the previous tool. Check the usage with:
pcl_mesh_sampling -h
Getting partial views
Tool
If you need to get a collection of partial snapshots of the model, PCL has also a tool for that. It is the virtual equivalent of using one of those rotating tables I told you about. It works just like pcl_mesh2pcd, but instead of composing all renders into a single file, it saves each one to its own.
pcl_virtual_scanner <input.ply>
Inside a directory, 42 .pcd files will be generated. You can check the usage by invoking it with no parameters; there are a couple of interesting options to add noise to the clouds, in order to better simulate the input from sensors like Kinect. If you need something more flexible (like a custom amount of snapshots, as the program does not let you change the number), see the next section.
Code
You can customize the snapshot generation process by making direct use of the "RenderViewsTesselatedSphere" class, which is similar to what the previous tool uses internally. The code is the following:
#include <pcl/io/vtk_lib_io.h>
#include <vtkPolyDataMapper.h>
#include <pcl/apps/render_views_tesselated_sphere.h>
int
main(int argc, char** argv)
{
// Load the PLY model from a file.
vtkSmartPointer<vtkPLYReader> reader = vtkSmartPointer<vtkPLYReader>::New();
reader->SetFileName(argv[1]);
reader->Update();
// VTK is not exactly straightforward...
vtkSmartPointer < vtkPolyDataMapper > mapper = vtkSmartPointer<vtkPolyDataMapper>::New();
mapper->SetInputConnection(reader->GetOutputPort());
mapper->Update();
vtkSmartPointer<vtkPolyData> object = mapper->GetInput();
// Virtual scanner object.
pcl::apps::RenderViewsTesselatedSphere render_views;
render_views.addModelFromPolyData(object);
// Pixel width of the rendering window, it directly affects the snapshot file size.
render_views.setResolution(150);
// Horizontal FoV of the virtual camera.
render_views.setViewAngle(57.0f);
// If true, the resulting clouds of the snapshots will be organized.
render_views.setGenOrganized(true);
// How much to subdivide the icosahedron. Increasing this will result in a lot more snapshots.
render_views.setTesselationLevel(1);
// If true, the camera will be placed at the vertices of the triangles. If false, at the centers.
// This will affect the number of snapshots produced (if true, less will be made).
// True: 42 for level 1, 162 for level 2, 642 for level 3...
// False: 80 for level 1, 320 for level 2, 1280 for level 3...
render_views.setUseVertices(true);
// If true, the entropies (the amount of occlusions) will be computed for each snapshot (optional).
render_views.setComputeEntropies(true);
render_views.generateViews();
// Object for storing the rendered views.
std::vector<pcl::PointCloud<pcl::PointXYZ>::Ptr> views;
// Object for storing the poses, as 4x4 transformation matrices.
std::vector<Eigen::Matrix4f, Eigen::aligned_allocator<Eigen::Matrix4f> > poses;
// Object for storing the entropies (optional).
std::vector<float> entropies;
render_views.getViews(views);
render_views.getPoses(poses);
render_views.getEntropies(entropies);
}
At the end of the program, the generated views (as point clouds) and the corresponding poses (transformation matrices) get saved to those vectors, so you can use them as you like (e.g., saving the snapshots to .pcd files and the poses to text files). There is no API documentation available, if you want to know more, check the render_views_tesselated_sphere.h file inside PCL's source folder.
If you get a segmentation fault, check if the .ply file is in ASCII format (you should be able to open and read it with a text editor). If it is, convert it to binary .ply using MeshLab. This usually solves it.
- Input: 3D model, [Resolution], [View angle], [Camera constraints], [Organized], [Sphere size]. [Tesselation level], [Use vertices], [Compute entropies]
- Output: Snapshot clouds, Snapshot poses, [Snapshot entropies]
- Download
Local pipeline
The following sections enumerate and explain the steps that compose the object recognition pipeline when using local descriptors.
Keypoint extraction
In this step, we have to decide which points from the current cloud will be considered keypoints (and have the local descriptor computed for them). The main characteristics of a good keypoint detector are:
- Repeatability: there should be a good chance of the same points being chosen over several iterations, even when the scene is captured from a different angle.
- Distinctiveness: the chosen keypoints should be highly characterizing and descriptive. It should be easy to describe and match them.
Because the second one depends on the local descriptor being used (and how the feature is computed), a different keypoint detection technique would have to be implemented for each. Another simple alternative is to perform downsampling on the cloud, and use all remaining points. This can be done easily with downsampling. Follow the link to find a code snippet that you can adapt to use in your system. You can also use the NARF keypoint detector.
ISS
Another keypoint detector available in PCL is the ISS (Intrinsic Shape Signatures) one. ISS is a local descriptor presented in 2009, which creates a view-independent signature of the local surface patch. An algorithm for choosing keypoints was also included to better fit the descriptor. The algorithm scans the surfaces and chooses only points with large variations in the principal direction (the shape of the surface), which are ideal for keypoints.
You can use the associated PCL class this way:
#include <pcl/io/pcd_io.h>
#include <pcl/keypoints/iss_3d.h>
// This function by Tommaso Cavallari and Federico Tombari, taken from the tutorial
// http://pointclouds.org/documentation/tutorials/correspondence_grouping.php
double
computeCloudResolution(const pcl::PointCloud<pcl::PointXYZ>::ConstPtr& cloud)
{
double resolution = 0.0;
int numberOfPoints = 0;
int nres;
std::vector<int> indices(2);
std::vector<float> squaredDistances(2);
pcl::search::KdTree<pcl::PointXYZ> tree;
tree.setInputCloud(cloud);
for (size_t i = 0; i < cloud->size(); ++i)
{
if (! pcl_isfinite((*cloud)[i].x))
continue;
// Considering the second neighbor since the first is the point itself.
nres = tree.nearestKSearch(i, 2, indices, squaredDistances);
if (nres == 2)
{
resolution += sqrt(squaredDistances[1]);
++numberOfPoints;
}
}
if (numberOfPoints != 0)
resolution /= numberOfPoints;
return resolution;
}
int
main(int argc, char** argv)
{
// Objects for storing the point cloud and the keypoints.
pcl::PointCloud<pcl::PointXYZ>::Ptr cloud(new pcl::PointCloud<pcl::PointXYZ>);
pcl::PointCloud<pcl::PointXYZ>::Ptr keypoints(new pcl::PointCloud<pcl::PointXYZ>);
// Read a PCD file from disk.
if (pcl::io::loadPCDFile<pcl::PointXYZ>(argv[1], *cloud) != 0)
{
return -1;
}
// ISS keypoint detector object.
pcl::ISSKeypoint3D<pcl::PointXYZ, pcl::PointXYZ> detector;
detector.setInputCloud(cloud);
pcl::search::KdTree<pcl::PointXYZ>::Ptr kdtree(new pcl::search::KdTree<pcl::PointXYZ>);
detector.setSearchMethod(kdtree);
double resolution = computeCloudResolution(cloud);
// Set the radius of the spherical neighborhood used to compute the scatter matrix.
detector.setSalientRadius(6 * resolution);
// Set the radius for the application of the non maxima supression algorithm.
detector.setNonMaxRadius(4 * resolution);
// Set the minimum number of neighbors that has to be found while applying the non maxima suppression algorithm.
detector.setMinNeighbors(5);
// Set the upper bound on the ratio between the second and the first eigenvalue.
detector.setThreshold21(0.975);
// Set the upper bound on the ratio between the third and the second eigenvalue.
detector.setThreshold32(0.975);
// Set the number of prpcessing threads to use. 0 sets it to automatic.
detector.setNumberOfThreads(4);
detector.compute(*keypoints);
}
- Input: Points, Search method, Salient radius, Non maxima radius, Minimum neighbors, Eigenvalues thresholds, [Number of threads]
- Output: Keypoints
- Publication:
- Intrinsic shape signatures: A shape descriptor for 3D object recognition (requires IEEE Xplore subscription) (Yu Zhong, 2009)
- API: pcl::ISSKeypoint3D
- Download
Computing descriptors
The next step is to compute the desired local descriptor(s) for every keypoint. The input parameters vary from one to another, and their efectiveness depends on the scene we are capturing and the objects we are working with, so I can not give you an ideal solution. Check the list of available descriptors, choose the one you deem best, and study the original publication to understand how it works and how the computation can be tuned to your needs, with the use of parameters (and try to understand how changing them affects the output). Refer to the given code snippets for each.
Matching
After the local descriptor has been computed for every keypoint in the cloud, we have to match them, finding correspondences with the ones we have stored in our object database. For this, a search structure like a k-d tree can be used to perform a nearest neighbor search, retrieving the Euclidean distances between descriptors (and optionally, enforcing a maximum distance value as a threshold). Every descriptor in the scene should be matched against the descriptors of every model in the database, because this accounts for the presence of multiple instances of the model, which would not be recognized if we did it the other way around.
The following code sample shows how to find all point correspondences between the cloud and a model:
#include <pcl/io/pcd_io.h>
#include <pcl/features/normal_3d.h>
#include <pcl/features/shot.h>
#include <iostream>
int
main(int argc, char** argv)
{
// Object for storing the SHOT descriptors for the scene.
pcl::PointCloud<pcl::SHOT352>::Ptr sceneDescriptors(new pcl::PointCloud<pcl::SHOT352>());
// Object for storing the SHOT descriptors for the model.
pcl::PointCloud<pcl::SHOT352>::Ptr modelDescriptors(new pcl::PointCloud<pcl::SHOT352>());
// Read the already computed descriptors from disk.
if (pcl::io::loadPCDFile<pcl::SHOT352>(argv[1], *sceneDescriptors) != 0)
{
return -1;
}
if (pcl::io::loadPCDFile<pcl::SHOT352>(argv[2], *modelDescriptors) != 0)
{
return -1;
}
// A kd-tree object that uses the FLANN library for fast search of nearest neighbors.
pcl::KdTreeFLANN<pcl::SHOT352> matching;
matching.setInputCloud(modelDescriptors);
// A Correspondence object stores the indices of the query and the match,
// and the distance/weight.
pcl::CorrespondencesPtr correspondences(new pcl::Correspondences());
// Check every descriptor computed for the scene.
for (size_t i = 0; i < sceneDescriptors->size(); ++i)
{
std::vector<int> neighbors(1);
std::vector<float> squaredDistances(1);
// Ignore NaNs.
if (pcl_isfinite(sceneDescriptors->at(i).descriptor[0]))
{
// Find the nearest neighbor (in descriptor space)...
int neighborCount = matching.nearestKSearch(sceneDescriptors->at(i), 1, neighbors, squaredDistances);
// ...and add a new correspondence if the distance is less than a threshold
// (SHOT distances are between 0 and 1, other descriptors use different metrics).
if (neighborCount == 1 && squaredDistances[0] < 0.25f)
{
pcl::Correspondence correspondence(neighbors[0], static_cast<int>(i), squaredDistances[0]);
correspondences->push_back(correspondence);
}
}
}
std::cout << "Found " << correspondences->size() << " correspondences." << std::endl;
}
Correspondence grouping
Right now, all we have is a list of correspondences between keypoints in the scene and keypoints from some object(s) in the database. This does not necessarily mean that a given object is present in the scene. Consider this example: a box with two keypoints located on opposite corners. The distance between the points is known. The matching stage has found two keypoints in the scene with very similar descriptors, but as it turns out, the distance between them is way different. Unless we are taking non-rigid transformations into account (such as scaling), then we have to discard the idea that those points belong to the object.
This check can be implemented with an additional step called correspondence grouping. Like the name states, it groups correspondences that are geometrically consistent (for the given object model) in clusters, and discards the ones that do not. Rotations and translations are permitted, but anything other than that will not meet the criteria. Also, as a minimum of 3 correspondences are required for retrieving the 6 DoF pose, groups with less than that can be ignored.
PCL offers a couple of classes to perform correspondence grouping.
Geometric consistency
This is the simplest method. It just iterates over all feature correspondences not yet grouped, and adds them to the current subset if they are geometrically consistent. The set resolution can be tuned to make the algorithm more or less forgiving when enforcing the consistency. For every subset (which should correspond with an instance of the model), this PCL class also computes the transformation (rotation and translation), making the next step (pose estimation) unnecessary.
#include <pcl/io/pcd_io.h>
#include <pcl/correspondence.h>
#include <pcl/recognition/cg/geometric_consistency.h>
#include <iostream>
int
main(int argc, char** argv)
{
// Objects for storing the keypoints of the scene and the model.
pcl::PointCloud<pcl::PointXYZ>::Ptr sceneKeypoints(new pcl::PointCloud<pcl::PointXYZ>);
pcl::PointCloud<pcl::PointXYZ>::Ptr modelKeypoints(new pcl::PointCloud<pcl::PointXYZ>);
// Objects for storing the unclustered and clustered correspondences.
pcl::CorrespondencesPtr correspondences(new pcl::Correspondences());
std::vector<pcl::Correspondences> clusteredCorrespondences;
// Object for storing the transformations (rotation plus translation).
std::vector<Eigen::Matrix4f, Eigen::aligned_allocator<Eigen::Matrix4f> > transformations;
// Read the keypoints from disk.
if (pcl::io::loadPCDFile<pcl::PointXYZ>(argv[1], *sceneKeypoints) != 0)
{
return -1;
}
if (pcl::io::loadPCDFile<pcl::PointXYZ>(argv[2], *modelKeypoints) != 0)
{
return -1;
}
// Note: here you would compute the correspondences.
// It has been omitted here for simplicity.
// Object for correspondence grouping.
pcl::GeometricConsistencyGrouping<pcl::PointXYZ, pcl::PointXYZ> grouping;
grouping.setSceneCloud(sceneKeypoints);
grouping.setInputCloud(modelKeypoints);
grouping.setModelSceneCorrespondences(correspondences);
// Minimum cluster size. Default is 3 (as at least 3 correspondences
// are needed to compute the 6 DoF pose).
grouping.setGCThreshold(3);
// Resolution of the consensus set used to cluster correspondences together,
// in metric units. Default is 1.0.
grouping.setGCSize(0.01);
grouping.recognize(transformations, clusteredCorrespondences);
std::cout << "Model instances found: " << transformations.size() << std::endl << std::endl;
for (size_t i = 0; i < transformations.size(); i++)
{
std::cout << "Instance " << (i + 1) << ":" << std::endl;
std::cout << "\tHas " << clusteredCorrespondences[i].size() << " correspondences." << std::endl << std::endl;
Eigen::Matrix3f rotation = transformations[i].block<3, 3>(0, 0);
Eigen::Vector3f translation = transformations[i].block<3, 1>(0, 3);
printf("\t\t | %6.3f %6.3f %6.3f | \n", rotation(0, 0), rotation(0, 1), rotation(0, 2));
printf("\t\tR = | %6.3f %6.3f %6.3f | \n", rotation(1, 0), rotation(1, 1), rotation(1, 2));
printf("\t\t | %6.3f %6.3f %6.3f | \n", rotation(2, 0), rotation(2, 1), rotation(2, 2));
std::cout << std::endl;
printf("\t\tt = < %0.3f, %0.3f, %0.3f >\n", translation(0), translation(1), translation(2));
}
}
If you do not need to compute the transformations (or prefer to do it later in its own step), you can use the "cluster()" function instead of the "recognize()" one, which only takes one parameter (the object for the clustered correspondences).
- Input: Points (model), Points (scene), Correspondences, Minimum cluster size, Consensus set resolution
- Output: Clustered correspondences, [Transformation]
- Tutorial: 3D Object Recognition based on Correspondence Grouping
- API: pcl::GeometricConsistencyGrouping
- Download
Hough voting
This method is a bit more complex. It uses a technique known as Hough transform, which was originally devised to perform line detection on 2D images, though it was later expanded to work with arbitrary shapes or higher dimensions. The method works with a voting procedure, with votes being cast on candidates that are found to be better suitable. If enough votes are accumulated for a given position in the space, then the shape is considered "found" there and its parameters are retrieved.
The voting procedure is carried out in a parameter space, which would have 6 dimensions in case a full pose needed to be estimated (rotation plus translation). Because that would be computationally expensive, the method implemented in PCL uses only a 3D Hough space, and employs local Reference Frames (RFs) to account for the remaining 3 DoF. Its computation can then be divided in two steps.
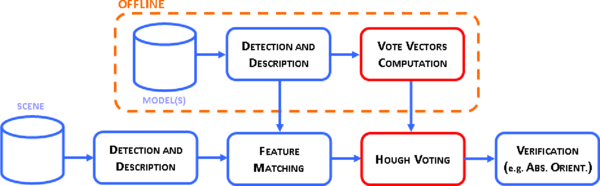
Computing Reference Frames
For every pair of correspondences, a local Reference Frame must be computed to retrieve the pose later. PCL offers a class that implements the BOrder Aware Repeatable Directions (BOARD) algorithm.
#include <pcl/io/pcd_io.h>
#include <pcl/features/board.h>
int
main(int argc, char** argv)
{
// Objects for storing the point clouds.
pcl::PointCloud<pcl::PointXYZ>::Ptr sceneCloud(new pcl::PointCloud<pcl::PointXYZ>);
pcl::PointCloud<pcl::PointXYZ>::Ptr modelCloud(new pcl::PointCloud<pcl::PointXYZ>);
// Objects for storing the normals.
pcl::PointCloud<pcl::Normal>::Ptr sceneNormals(new pcl::PointCloud<pcl::Normal>);
pcl::PointCloud<pcl::Normal>::Ptr modelNormals(new pcl::PointCloud<pcl::Normal>);
// Objects for storing the keypoints.
pcl::PointCloud<pcl::PointXYZ>::Ptr sceneKeypoints(new pcl::PointCloud<pcl::PointXYZ>);
pcl::PointCloud<pcl::PointXYZ>::Ptr modelKeypoints(new pcl::PointCloud<pcl::PointXYZ>);
// Objects for storing the Reference Frames.
pcl::PointCloud<pcl::ReferenceFrame>::Ptr sceneRF(new pcl::PointCloud<pcl::ReferenceFrame>);
pcl::PointCloud<pcl::ReferenceFrame>::Ptr modelRF(new pcl::PointCloud<pcl::ReferenceFrame>);
// Read the scene and model clouds from disk.
if (pcl::io::loadPCDFile<pcl::PointXYZ>(argv[1], *sceneCloud) != 0)
{
return -1;
}
if (pcl::io::loadPCDFile<pcl::PointXYZ>(argv[2], *modelCloud) != 0)
{
return -1;
}
// Note: here you would estimate the normals for the whole cloud, and choose
// the keypoints. It has been omitted here for simplicity.
// BOARD RF estimation object.
pcl::BOARDLocalReferenceFrameEstimation<pcl::PointXYZ, pcl::Normal, pcl::ReferenceFrame> rf;
// Search radius (maximum distance of the points used to estimate the X and Y axes
// of the BOARD Reference Frame for a given point).
rf.setRadiusSearch(0.02f);
// Check if support is complete, or has missing regions because it is too close to mesh borders.
rf.setFindHoles(true);
rf.setInputCloud(sceneKeypoints);
rf.setInputNormals(sceneNormals);
rf.setSearchSurface(sceneCloud);
rf.compute(*sceneRF);
rf.setInputCloud(modelKeypoints);
rf.setInputNormals(modelNormals);
rf.setSearchSurface(modelCloud);
rf.compute(*modelRF);
}
Be sure to check the API reference because the BOARD estimation object has many more parameters to tune.
- Input: Points, Normals, Search radius, [Tangent radius], [Margin array size], [Margin threshold], [Find holes], [Hole size threshold], [Steepness threshold]
- Output: Reference Frames
- Tutorial: 3D Object Recognition based on Correspondence Grouping
- Publication:
- On the repeatability of the local reference frame for partial shape matching (Alioscia Petrelli and Luigi Di Stefano, 2011)
- API: pcl::BOARDLocalReferenceFrameEstimation
- Download
Clustering
#include <pcl/io/pcd_io.h>
#include <pcl/recognition/cg/hough_3d.h>
#include <iostream>
int
main(int argc, char** argv)
{
// Objects for storing the keypoints of the scene and the model.
pcl::PointCloud<pcl::PointXYZ>::Ptr sceneKeypoints(new pcl::PointCloud<pcl::PointXYZ>);
pcl::PointCloud<pcl::PointXYZ>::Ptr modelKeypoints(new pcl::PointCloud<pcl::PointXYZ>);
// Objects for storing the Reference Frames.
pcl::PointCloud<pcl::ReferenceFrame>::Ptr sceneRF(new pcl::PointCloud<pcl::ReferenceFrame>);
pcl::PointCloud<pcl::ReferenceFrame>::Ptr modelRF(new pcl::PointCloud<pcl::ReferenceFrame>);
// Objects for storing the unclustered and clustered correspondences.
pcl::CorrespondencesPtr correspondences(new pcl::Correspondences());
std::vector<pcl::Correspondences> clusteredCorrespondences;
// Object for storing the transformations (rotation plus translation).
std::vector<Eigen::Matrix4f, Eigen::aligned_allocator<Eigen::Matrix4f> > transformations;
// Read the keypoints from disk.
if (pcl::io::loadPCDFile<pcl::PointXYZ>(argv[1], *sceneKeypoints) != 0)
{
return -1;
}
if (pcl::io::loadPCDFile<pcl::PointXYZ>(argv[2], *modelKeypoints) != 0)
{
return -1;
}
// Note: here you would compute the correspondences and the Reference Frames.
// It has been omitted here for simplicity.
// Object for correspondence grouping.
pcl::Hough3DGrouping<pcl::PointXYZ, pcl::PointXYZ, pcl::ReferenceFrame, pcl::ReferenceFrame> grouping;
grouping.setInputCloud(modelKeypoints);
grouping.setInputRf(modelRF);
grouping.setSceneCloud(sceneKeypoints);
grouping.setSceneRf(sceneRF);
grouping.setModelSceneCorrespondences(correspondences);
// Minimum cluster size. Default is 3 (as at least 3 correspondences
// are needed to compute the 6 DoF pose).
grouping.setHoughThreshold(3);
// Size of each bin in the Hough space.
grouping.setHoughBinSize(3);
// If true, the vote casting procedure will interpolate the score
// between neighboring bins in the Hough space.
grouping.setUseInterpolation(true);
// If true, the vote casting procedure will use the correspondence's
// weighted distance to compute the Hough voting score.
grouping.setUseDistanceWeight(false);
grouping.recognize(transformations, clusteredCorrespondences);
std::cout << "Model instances found: " << transformations.size() << std::endl << std::endl;
for (size_t i = 0; i < transformations.size(); i++)
{
std::cout << "Instance " << (i + 1) << ":" << std::endl;
std::cout << "\tHas " << clusteredCorrespondences[i].size() << " correspondences." << std::endl << std::endl;
Eigen::Matrix3f rotation = transformations[i].block<3, 3>(0, 0);
Eigen::Vector3f translation = transformations[i].block<3, 1>(0, 3);
printf("\t\t | %6.3f %6.3f %6.3f | \n", rotation(0, 0), rotation(0, 1), rotation(0, 2));
printf("\t\tR = | %6.3f %6.3f %6.3f | \n", rotation(1, 0), rotation(1, 1), rotation(1, 2));
printf("\t\t | %6.3f %6.3f %6.3f | \n", rotation(2, 0), rotation(2, 1), rotation(2, 2));
std::cout << std::endl;
printf("\t\tt = < %0.3f, %0.3f, %0.3f >\n", translation(0), translation(1), translation(2));
}
}
If you do not give the Reference Frames to the Hough grouping object, it will calculate them itself, but then you need to specify additional parameters. See the API for more details. Also, you can "cluster()" instead of "recognize()" if you want to skip the pose estimation now.
- Input: Points (model), Points (scene), Correspondences, Reference Frames (model), Reference Frames (scene), Hough bin size, Hough threshold, [Use distance weight], [Use interpolation]
- Output: Clustered correspondences, [Transformation]
- Tutorial: 3D Object Recognition based on Correspondence Grouping
- Publication:
- Object recognition in 3D scenes with occlusions and clutter by Hough voting (Federico Tombari and Luigi Di Stefano, 2010)
- API: pcl::Hough3DGrouping
- Download
Pose estimation
The two correspondence grouping classes included in PCL already compute the full pose, but you can do it manually if you want, or if you want to refine the results of the previous step (removing correspondences that are not compatible with the same 6 DoF pose). You can use a method like RANSAC (RANdom SAmple Consensus), to get the rotation and translation of the rigid transformation that best fits the correspondences of a model instance. In a previous tutorial we mentioned that there was a technique called feature-based registration which was an alternative to ICP, and this is it.
Of course, PCL has some classes for this. The one we are going to see adds a prerejection step to the RANSAC loop, in order to discard hypotheses that are probably wrong. To do this, the algorithm forms polygons with the points of the input sets, and then checks pose-invariant geometrical constraints. To be precise, it makes sure that the polygon edges are of similar length. You can let it run for a specified number of iterations (as, like you already know, RANSAC never actually converges), or set a threshold.
#include <pcl/io/pcd_io.h>
#include <pcl/features/shot.h>
#include <pcl/registration/sample_consensus_prerejective.h>
#include <iostream>
int
main(int argc, char** argv)
{
// Objects for storing the scene and the model.
pcl::PointCloud<pcl::PointXYZ>::Ptr scene(new pcl::PointCloud<pcl::PointXYZ>);
pcl::PointCloud<pcl::PointXYZ>::Ptr model(new pcl::PointCloud<pcl::PointXYZ>);
pcl::PointCloud<pcl::PointXYZ>::Ptr alignedModel(new pcl::PointCloud<pcl::PointXYZ>);
// Objects for storing the SHOT descriptors for the scene and model.
pcl::PointCloud<pcl::SHOT352>::Ptr sceneDescriptors(new pcl::PointCloud<pcl::SHOT352>());
pcl::PointCloud<pcl::SHOT352>::Ptr modelDescriptors(new pcl::PointCloud<pcl::SHOT352>());
// Read the clouds from disk.
if (pcl::io::loadPCDFile<pcl::PointXYZ>(argv[1], *scene) != 0)
{
return -1;
}
if (pcl::io::loadPCDFile<pcl::PointXYZ>(argv[2], *model) != 0)
{
return -1;
}
// Note: here you would compute or load the descriptors for both
// the scene and the model. It has been omitted here for simplicity.
// Object for pose estimation.
pcl::SampleConsensusPrerejective<pcl::PointXYZ, pcl::PointXYZ, pcl::SHOT352> pose;
pose.setInputSource(model);
pose.setInputTarget(scene);
pose.setSourceFeatures(modelDescriptors);
pose.setTargetFeatures(sceneDescriptors);
// Instead of matching a descriptor with its nearest neighbor, choose randomly between
// the N closest ones, making it more robust to outliers, but increasing time.
pose.setCorrespondenceRandomness(2);
// Set the fraction (0-1) of inlier points required for accepting a transformation.
// At least this number of points will need to be aligned to accept a pose.
pose.setInlierFraction(0.25f);
// Set the number of samples to use during each iteration (minimum for 6 DoF is 3).
pose.setNumberOfSamples(3);
// Set the similarity threshold (0-1) between edge lengths of the polygons. The
// closer to 1, the more strict the rejector will be, probably discarding acceptable poses.
pose.setSimilarityThreshold(0.6f);
// Set the maximum distance threshold between two correspondent points in source and target.
// If the distance is larger, the points will be ignored in the alignment process.
pose.setMaxCorrespondenceDistance(0.01f);
pose.align(*alignedModel);
if (pose.hasConverged())
{
Eigen::Matrix4f transformation = pose.getFinalTransformation();
Eigen::Matrix3f rotation = transformation.block<3, 3>(0, 0);
Eigen::Vector3f translation = transformation.block<3, 1>(0, 3);
std::cout << "Transformation matrix:" << std::endl << std::endl;
printf("\t\t | %6.3f %6.3f %6.3f | \n", rotation(0, 0), rotation(0, 1), rotation(0, 2));
printf("\t\tR = | %6.3f %6.3f %6.3f | \n", rotation(1, 0), rotation(1, 1), rotation(1, 2));
printf("\t\t | %6.3f %6.3f %6.3f | \n", rotation(2, 0), rotation(2, 1), rotation(2, 2));
std::cout << std::endl;
printf("\t\tt = < %0.3f, %0.3f, %0.3f >\n", translation(0), translation(1), translation(2));
}
else std::cout << "Did not converge." << std::endl;
}
For an alternative, check the pcl::SampleConsensusModelRegistration or the pcl::SampleConsensusInitialAlignment classes.
- Input: Points (model), Points (scene), Descriptors (model), Descriptors (scene), [Correspondence randomness], [Inlier fraction], [Number of samples], [Similarity threshold]
- Output: Aligned model, [Transformation]
- Tutorials:
- Publication:
- Pose Estimation using Local Structure-Specific Shape and Appearance Context (Anders Glent Buch et al., 2013)
- API:
- Download
Global pipeline
Because of the way global descriptors work, the global pipeline for object recognition differs in some steps.
Segmentation
Unlike local descriptors, global ones understand the notion of object. They are not computed for single points, but for whole clusters. Because of this, the first step is to perform segmentation on the cloud in order to retrieve all objects. You can use any method you like from the ones available in PCL, as long as it works for you. Also, performing some kind of preprocessing is a good idea, like removing all clusters that are smaller or bigger than certain thresholds, which should be set according to the objects in the database. Yet another possibility is to perform plane segmentation to find any table(s) in the scene, and consider only clusters sitting on it (if we can assume there is a table, of course).
PCL provides the pcl::ExtractPolygonalPrismData class for the latter task. By giving a convex hull computed from the plane coefficients, it will extrude it a certain height to create a prism, and then give back all points that lie inside. This is the code:
#include <pcl/io/pcd_io.h>
#include <pcl/sample_consensus/method_types.h>
#include <pcl/sample_consensus/model_types.h>
#include <pcl/segmentation/sac_segmentation.h>
#include <pcl/filters/extract_indices.h>
#include <pcl/surface/convex_hull.h>
#include <pcl/segmentation/extract_polygonal_prism_data.h>
#include <pcl/visualization/cloud_viewer.h>
#include <iostream>
int
main(int argc, char** argv)
{
// Objects for storing the point clouds.
pcl::PointCloud<pcl::PointXYZ>::Ptr cloud(new pcl::PointCloud<pcl::PointXYZ>);
pcl::PointCloud<pcl::PointXYZ>::Ptr plane(new pcl::PointCloud<pcl::PointXYZ>);
pcl::PointCloud<pcl::PointXYZ>::Ptr convexHull(new pcl::PointCloud<pcl::PointXYZ>);
pcl::PointCloud<pcl::PointXYZ>::Ptr objects(new pcl::PointCloud<pcl::PointXYZ>);
// Read a PCD file from disk.
if (pcl::io::loadPCDFile<pcl::PointXYZ>(argv[1], *cloud) != 0)
{
return -1;
}
// Get the plane model, if present.
pcl::ModelCoefficients::Ptr coefficients(new pcl::ModelCoefficients);
pcl::SACSegmentation<pcl::PointXYZ> segmentation;
segmentation.setInputCloud(cloud);
segmentation.setModelType(pcl::SACMODEL_PLANE);
segmentation.setMethodType(pcl::SAC_RANSAC);
segmentation.setDistanceThreshold(0.01);
segmentation.setOptimizeCoefficients(true);
pcl::PointIndices::Ptr planeIndices(new pcl::PointIndices);
segmentation.segment(*planeIndices, *coefficients);
if (planeIndices->indices.size() == 0)
std::cout << "Could not find a plane in the scene." << std::endl;
else
{
// Copy the points of the plane to a new cloud.
pcl::ExtractIndices<pcl::PointXYZ> extract;
extract.setInputCloud(cloud);
extract.setIndices(planeIndices);
extract.filter(*plane);
// Retrieve the convex hull.
pcl::ConvexHull<pcl::PointXYZ> hull;
hull.setInputCloud(plane);
// Make sure that the resulting hull is bidimensional.
hull.setDimension(2);
hull.reconstruct(*convexHull);
// Redundant check.
if (hull.getDimension() == 2)
{
// Prism object.
pcl::ExtractPolygonalPrismData<pcl::PointXYZ> prism;
prism.setInputCloud(cloud);
prism.setInputPlanarHull(convexHull);
// First parameter: minimum Z value. Set to 0, segments objects lying on the plane (can be negative).
// Second parameter: maximum Z value, set to 10cm. Tune it according to the height of the objects you expect.
prism.setHeightLimits(0.0f, 0.1f);
pcl::PointIndices::Ptr objectIndices(new pcl::PointIndices);
prism.segment(*objectIndices);
// Get and show all points retrieved by the hull.
extract.setIndices(objectIndices);
extract.filter(*objects);
pcl::visualization::CloudViewer viewerObjects("Objects on table");
viewerObjects.showCloud(objects);
while (!viewerObjects.wasStopped())
{
// Do nothing but wait.
}
}
else std::cout << "The chosen hull is not planar." << std::endl;
}
}
If you get some residual points of the table in the output, try increasing the minimum Z value a bit.
- Input: Points, Convex hull, Height limits, [Dimensionality]
- Output: Point indices
- API: pcl::ExtractPolygonalPrismData
- Download
Computing descriptors
For every cluster that has survived the previous step, a global descriptor must be computed. Most only output one histogram (except for CVFH and derivatives), so the database will be smaller than with local descriptors. Check the list and choose the one that fits you best.
CRH
In a previous article about global descriptors we talked about how they were invariant to the camera roll angle. When CVFH was presented, the computation of a Camera Roll Histogram (CRH) was proposed to overcome this. The CRH should be computed and stored next to the global descriptor (VFH, CVFH...) for its use in a later phase, the pose estimation.
The CRH is computed as follows: for every point, its normal is projected onto a plane orthogonal to the vector given by the camera center and the centroid of the cluster (VFH) or region (CVFH). Then, the angle of the projected normal to the up vector of the camera is computed and added to the histogram, which has 90 bins (for a resolution of 4º). The projected normals are weighted by their magnitudes to reduce the effect of input noise. Check the paper for details.
You can compute it using PCL, of course:
#include <pcl/io/pcd_io.h>
#include <pcl/features/normal_3d.h>
#include <pcl/common/centroid.h>
#include <pcl/features/crh.h>
// A handy typedef.
typedef pcl::Histogram<90> CRH90;
int
main(int argc, char** argv)
{
// Cloud for storing the object.
pcl::PointCloud<pcl::PointXYZ>::Ptr object(new pcl::PointCloud<pcl::PointXYZ>);
// Object for storing the normals.
pcl::PointCloud<pcl::Normal>::Ptr normals(new pcl::PointCloud<pcl::Normal>);
// Object for storing the CRH.
pcl::PointCloud<CRH90>::Ptr histogram(new pcl::PointCloud<CRH90>);
// Note: this cloud file should contain a snapshot of the object. Remember
// that you need to compute a CRH for every VFH or CVFH descriptor that you
// are going to have (that is, one for every snapshot).
// Read a PCD file from disk.
if (pcl::io::loadPCDFile<pcl::PointXYZ>(argv[1], *object) != 0)
{
return -1;
}
// Estimate the normals.
pcl::NormalEstimation<pcl::PointXYZ, pcl::Normal> normalEstimation;
normalEstimation.setInputCloud(object);
normalEstimation.setRadiusSearch(0.03);
pcl::search::KdTree<pcl::PointXYZ>::Ptr kdtree(new pcl::search::KdTree<pcl::PointXYZ>);
normalEstimation.setSearchMethod(kdtree);
normalEstimation.compute(*normals);
// CRH estimation object.
pcl::CRHEstimation<pcl::PointXYZ, pcl::Normal, CRH90> crh;
crh.setInputCloud(object);
crh.setInputNormals(normals);
Eigen::Vector4f centroid;
pcl::compute3DCentroid(*object, centroid);
crh.setCentroid(centroid);
// Compute the CRH.
crh.compute(*histogram);
}
- Input: Points (cluster), Normals, Centroid
- Output: Camera roll histogram
- Publication:
- CAD-Model Recognition and 6DOF Pose Estimation Using 3D Cues (requires IEEE Xplore subscription) (Aitor Aldoma et al., 2011)
- API: pcl::CRHEstimation
- Download
Matching
Like with the local pipeline, once all the descriptors have been computed we have to perform a search for their nearest neighbors in the database. The Manhattan or L1 distance is recommended over the Euclidean or L2 one because it is more robust to occlusions.
Usually, more than one neighbor is retrieved, which means that the found object's pose is somewhere between the two. The next steps can help improving the accuraccy of the estimation.
Pose estimation
The object's position can be determined by computing and aligning the centroids of the clusters (the one in the scene, and the one in the snapshot). For the rotation, we must use the viewpoint information encoded in the descriptor (you can check the ground truth pose information that was saved with the matched snapshot). This still leaves the roll angle unresolved, as global descriptors are invariant to it. Now is when the Camera Roll Histogram (CRH) that we should have stored next to the descriptor comes in handy. The angle can be obtained by aligning the current CRH with the stored one, completing the 6 DoF pose estimation.
CRH
This is the code for retrieving the roll angle using CRH:
#include <pcl/io/pcd_io.h>
#include <pcl/common/centroid.h>
#include <pcl/features/crh.h>
#include <pcl/recognition/crh_alignment.h>
#include <iostream>
#include <vector>
// A handy typedef.
typedef pcl::Histogram<90> CRH90;
int
main(int argc, char** argv)
{
// Clouds for storing the object's cluster and view.
pcl::PointCloud<pcl::PointXYZ>::Ptr viewCloud(new pcl::PointCloud<pcl::PointXYZ>);
pcl::PointCloud<pcl::PointXYZ>::Ptr clusterCloud(new pcl::PointCloud<pcl::PointXYZ>);
// Objects for storing the CRHs of both.
pcl::PointCloud<CRH90>::Ptr viewCRH(new pcl::PointCloud<CRH90>);
pcl::PointCloud<CRH90>::Ptr clusterCRH(new pcl::PointCloud<CRH90>);
// Objects for storing the centroids.
Eigen::Vector4f viewCentroid;
Eigen::Vector4f clusterCentroid;
// Read the trained view and the scene cluster from disk.
if (pcl::io::loadPCDFile<pcl::PointXYZ>(argv[1], *viewCloud) != 0)
{
return -1;
}
if (pcl::io::loadPCDFile<pcl::PointXYZ>(argv[2], *clusterCloud) != 0)
{
return -1;
}
// Note: here you would compute the CRHs and centroids of both clusters.
// It has been omitted here for simplicity.
// CRH alignment object.
pcl::CRHAlignment<pcl::PointXYZ, 90> alignment;
alignment.setInputAndTargetView(clusterCloud, viewCloud);
// CRHAlignment works with Vector3f, not Vector4f.
Eigen::Vector3f viewCentroid3f(viewCentroid[0], viewCentroid[1], viewCentroid[2]);
Eigen::Vector3f clusterCentroid3f(clusterCentroid[0], clusterCentroid[1], clusterCentroid[2]);
alignment.setInputAndTargetCentroids(clusterCentroid3f, viewCentroid3f);
// Compute the roll angle(s).
std::vector<float> angles;
alignment.computeRollAngle(*clusterCRH, *viewCRH, angles);
if (angles.size() > 0)
{
std::cout << "List of angles where the histograms correlate:" << std::endl;
for (int i = 0; i < angles.size(); i++)
{
std::cout << "\t" << angles.at(i) << " degrees." << std::endl;
}
}
}
This will return a list of most probable roll angles. If you want, you can instead use the align() function, which calls "computeRollAngle()" internally. It will return a list of transformations that include the translation (computed from the difference between the centroid's coordinates) and the roll angles, but not the yaw or pitch (you have to get those with pose estimation).
- Input: Points (input and target), Centroids (input and target), CRHs (input and target)
- Output: Roll angles, [Transformations]
- Publication:
- CAD-Model Recognition and 6DOF Pose Estimation Using 3D Cues (requires IEEE Xplore subscription) (Aitor Aldoma et al., 2011)
- API: pcl::CRHAlignment
- Download
Postprocessing
The pipelines, as they are, should already return a decent result, but we can implement optional steps to improve the accuraccy of the pose estimation, and decrease the probability of a false positive.
Pose refinement
The 6 DoF pose estimation can be refined with the use of the Iterative Closest Point (ICP) algorithm. It will try to continuously realign the clouds to improve the transformation, until the termination condition is met (maximum iterations, and distance or error threshold). This is identical to performing registration, only between input and model.
Hypothesis verification
(Work in progress)
Go to root: PhD-3D-Object-Tracking
Links to articles:
PCL/OpenNI tutorial 0: The very basics
PCL/OpenNI tutorial 1: Installing and testing
PCL/OpenNI tutorial 2: Cloud processing (basic)
PCL/OpenNI tutorial 3: Cloud processing (advanced)
PCL/OpenNI tutorial 4: 3D object recognition (descriptors)
PCL/OpenNI tutorial 5: 3D object recognition (pipeline)